Branislav Bajat
Dr. (Mr.) Branislav Bajat is a professor in the Department of Geodesy and Geoinformatics, Faculty of Civil Engineering, University of Belgrade. His main research area is the application of novel spatial and spatio-temporal statistical methodologies in geosciences and environmental disciplines. His experience includes the application multi- and interdisciplinary research on spatial knowledge in environmental sciences; GI research based on commercial and open source tools; application of data mining and machine learning techniques to spatio-temporal data.
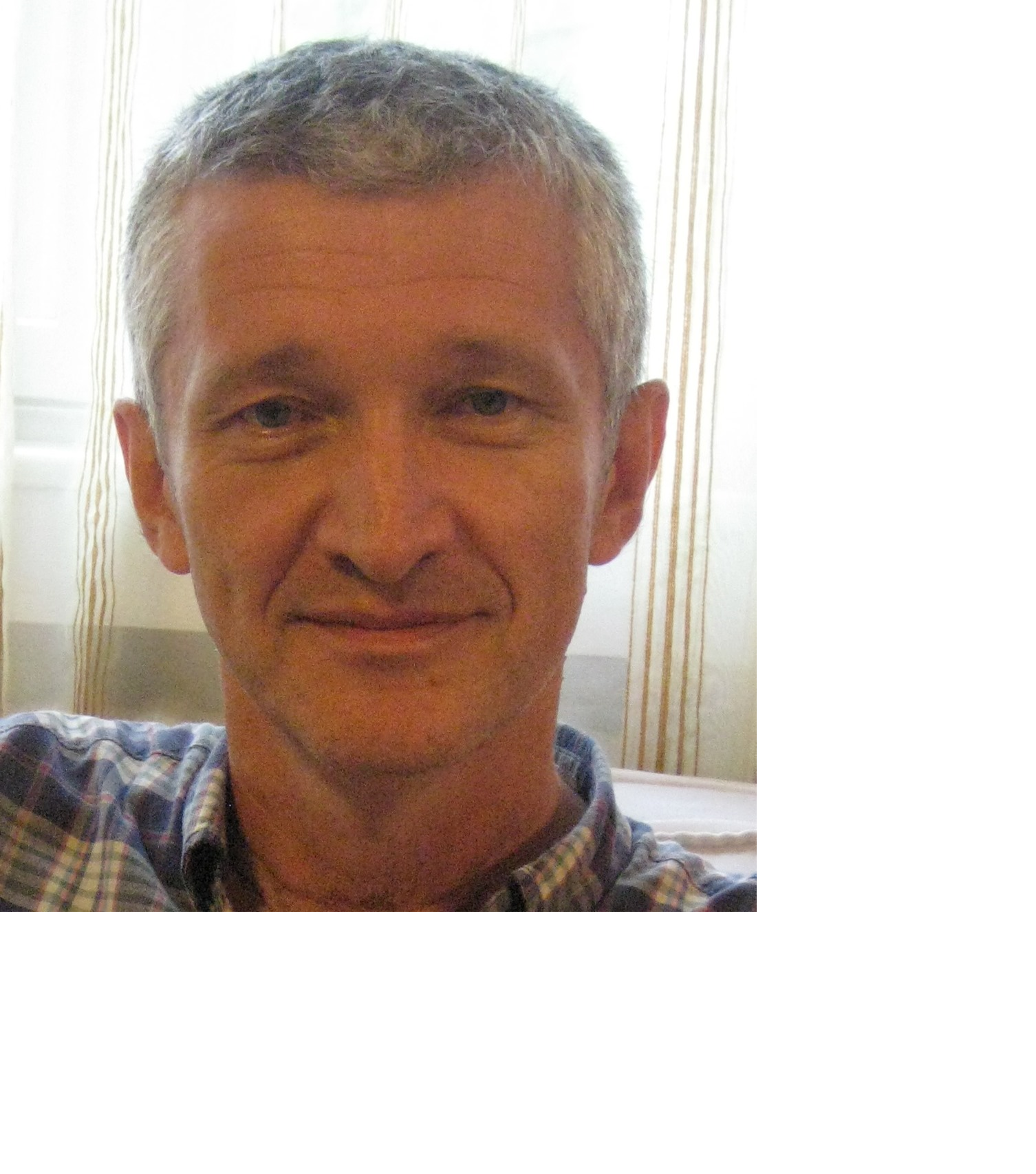
Sessions
The use of AI in agriculture is a trend all over the world; however, in Serbia it should be especially important because agriculture is one of the crucial sectors of Serbian economy. This project will be an important step forward in the application of a wide range of relevant data generated on a daily basis and offering a huge potential for improving agricultural production and developing the concept of smart and regenerative agriculture.
The CERES project deals with the development of tools based on Artificial Intelligence (AI) algorithms intended to support agricultural production and Carbon Farming practices which result in carbon sequestration in soil and combat climate change. The idea of the project is to create models which would use big geospatial data from various sources – optical and radar satellite images (Copernicus missions), soil related data (in-situ, LandGIS, SoilGrids, LUCAS), accurate meteorological data and textual data available on internet portals intended for agriculture – to automatically generate new information which will help make timely and correct decisions in agriculture. The quantity of publicly available data has been growing at a massive amount and will continue to do so in the near future. Given the limited amount of the available for Serbia, a distinct challenge will be how to leverage the potential of existing global data and models made for wider areas to improve the local models. Considering the existence of distribution (domain) discrepancy between the global and local data, the main challenge is to discover the proper approach of Domain Adaptation (DA), the transfer learning technique, in which the system aims to adapt the knowledge learned from source domain, and apply it to another related domain with the aim to compensate the “lack of data”. Considering various sources of relevant data on global scales, Multi-source DA (MDA), an additional form of DA, which involves the existence of more than one source domain should be adapt to compensate “lack of data”.
In addition, this work will present the progress in developing on the other approaches related to projects aims, including the models for early identification of discontinuity and other disturbance in crop growth, yield prediction as well as the estimation of soil organic carbon concentration in soil and identification of tillage activities.