Daniel Žížala
01/01/2008 – Present Soil scientist
Research Institute for Soil and Water Conservation, Prague (Czechia)
2017 – today: Head of Remote Sensing and Pedometrics Lab
GIS and Remote Sensing analysis, data analysis, data mining, pedometrics and digital soil mapping,
precision farming, map server and web map services administrator, project management (researcher
responsible for solving research projects and contracts), processing and analysis of aerial and satellite
images, Spatial database management and processing of the data, Soil erosion modelling
2012–2018 Ph.D.
Czech University of Life Science Prague, Prague (Czechia)
Program: Agricultural Special Sciences
Thesis titled “Mapping of soil organic carbon redistribution in eroded soils using remote sensing and
digital soil maping methods” It is study on a quantitative assessment of soil organic carbon
redistribution by soil erosion using progressive methods of survey particularly remote sensing
methods and digital soil mapping methods.
2002–2008 MSc.
Charles University in Prague, Prague (Czechia)
Program: Physical Geography and Geoecology
Thesis titled “Analysis of Genesis and Activity of Slope Deformation Běleč in Context of Evolution of
the Svratka River Valley between Doubravník and Borač”. It was geomorphological study on analysis
of landscape evolution of the Svratka River Valley on the western Moravia in context of rock slope
instability in Běleč. Geomorphological and morphotectonical methods was used for landscape
evolution analysis and dendrogeomorphological methods was used for slope deformation activity
analysis.
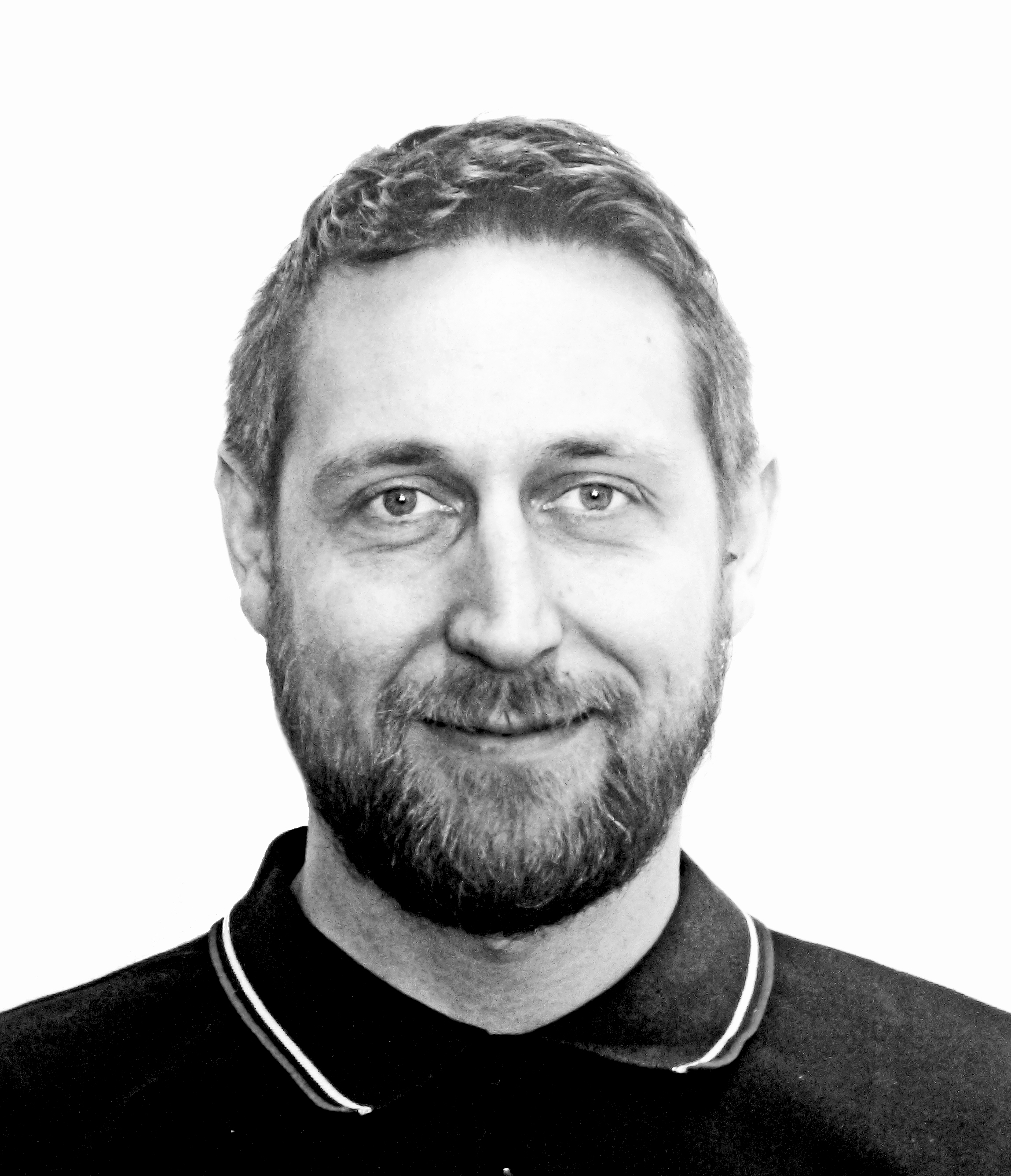
Sessions
The available satellite multispectral data from the European Copernicus programme allow, among other things, their use for the purpose of mapping soil properties. This can be done on images that capture the bare soil surface. Due to the fact that only a part of the land is free of vegetation cover at any one time, mapping can only be carried out over a limited area. For regional mapping, a spatio-temporal composite of satellite imagery is required from the time series of image data. Such a composite was created for the Czech Republic using Sentinel-2 data and Google Earth Engine. The bare soil composite was then used to predict soil properties (texture, carbon content, pH) using soil samples and machine learning methods. Specifically, the quantile random forest method was used to both predict the target variables and to determine prediction intervals at single image points. This resulted in a unique set of high spatial resolution maps of soil properties of Czech soils.